Probabilistic Relational Models
Principle Investigator: Prof. Dr. Ralf Möller
Research Associate: Dr. Tanya Braun
A probabilistic relational model (PRM) or a relational probability model is a model in which the probabilities are specified on the relations, independently of the actual individuals. Different individuals share the probability parameters.
Lifted Junction Tree Algorithm
We look at probabilistic relational formalisms where the domain objects are known. In these formalisms, standard approaches for inference use lifted variable elimination (LVE) to answer single queries, which leads to inefficiencies for multiple queries. To answer multiple queries efficiently, the well-known junction tree algorithm builds a cluster representation of the underlying model for faster query answering. To benefit from the idea behind junction trees in the relational setting, we have transferred the concept of lifting to the junction tree algorithm, presenting the lifted junction tree algorithm (LJT). LJT saves computations using a compact first-order cluster representation and LVE as a subroutine in its computations.
While before either the number of known objects has proven to limit the junction tree algorithm or the number of queries lead to inefficient repetition for LVE, LJT allows for efficient repeated inference by incorporating relational aspects of a model as well as avoiding duplicate calculations. In many settings, LJT even outperforms FOKC, another well-known algorithm for exact repeated inference. FOKC stands for first-order knowledge compilation, which solves a weighted first-order model counting problem by building a first-order circuit, in which FOKC computes weighted model counts.
So far, we have extended the original LJT
- to include the lifting tool of counting to lift even more computations,
- to identify and prevent unnecessary groundings through an additional merging step called fusion,
- to effectively handle evidence in a lifted manner, and
- to answer conjunctive queries that span more than one cluster.
We also work on a dynamic version of LJT (LDJT) to handle sequential data, e.g., in the form of time series. For more information, please refer to LDJT. We further apply the lifting idea to extend LVE, LJT, and LDJT
- to compute a most probable explanation (also known as total abduction), including safe MAP queries (partial abduction),
- to answer parameterised queries (compact form of a conjunctive query with isomorphic query terms) for a lifted query answering,
- to handle uncertain evidence (observations of events with probability p < 1.0), and
- to compute a maximum expected utility.
Not only LVE works as a subroutine. LJT allows for any inference algorithms as a subroutine as long as an algorithm fulfils certain requirements for evidence handling and message passing. The subroutine algorithm determines what type of queries LJT can answer. We have published a version of LJT that combines LVE and FOKC as subroutines into LJTKC.
Description of the Input Files for the JAIR Submission
Please find here a manuscript describing the input files used for the evaluation of LJT, LVE and FOKC as well as JT and VE:
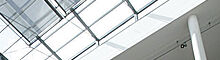
- Team
- Umut Çalıkyılmaz
- Rebecca von Engelhardt
- Björn Filter
- Nils Fußgänger
- Jinghua Groppe
- Sven Groppe
- Tobias Groth
- Mattis Hartwig
- Nils Wendel Heinrich
- Akasha Kessel
- Hanieh Khorashadizadeh
- Malte Luttermann
- Jörg-Uwe Meyer
- Jeannette Mödlhammer
- Nitin Nayak
- Simon Paasche
- Michael Preisig
- Nele Rußwinkel
- Simon Schiff
- Tim Schulz
- Thomas Sievers
- Tobias Winker
- Alumni